More than 20 years ago, fresh out of school in the 90s, I built artificial intelligence (AI) systems for a military contractor. I trained neural nets, used natural language processing to populate decision support systems, experimented with genetic algorithms, and refined support vector machines. In 2000 I pivoted to bioinformatics and genomics – mostly because I wanted to help people live longer, healthier lives rather than building algorithms to kill them ever more efficiently.
It’s taken a while for the public sector to catch up, but we’re finally here and I am all in on AI for genomics and precision medicine.
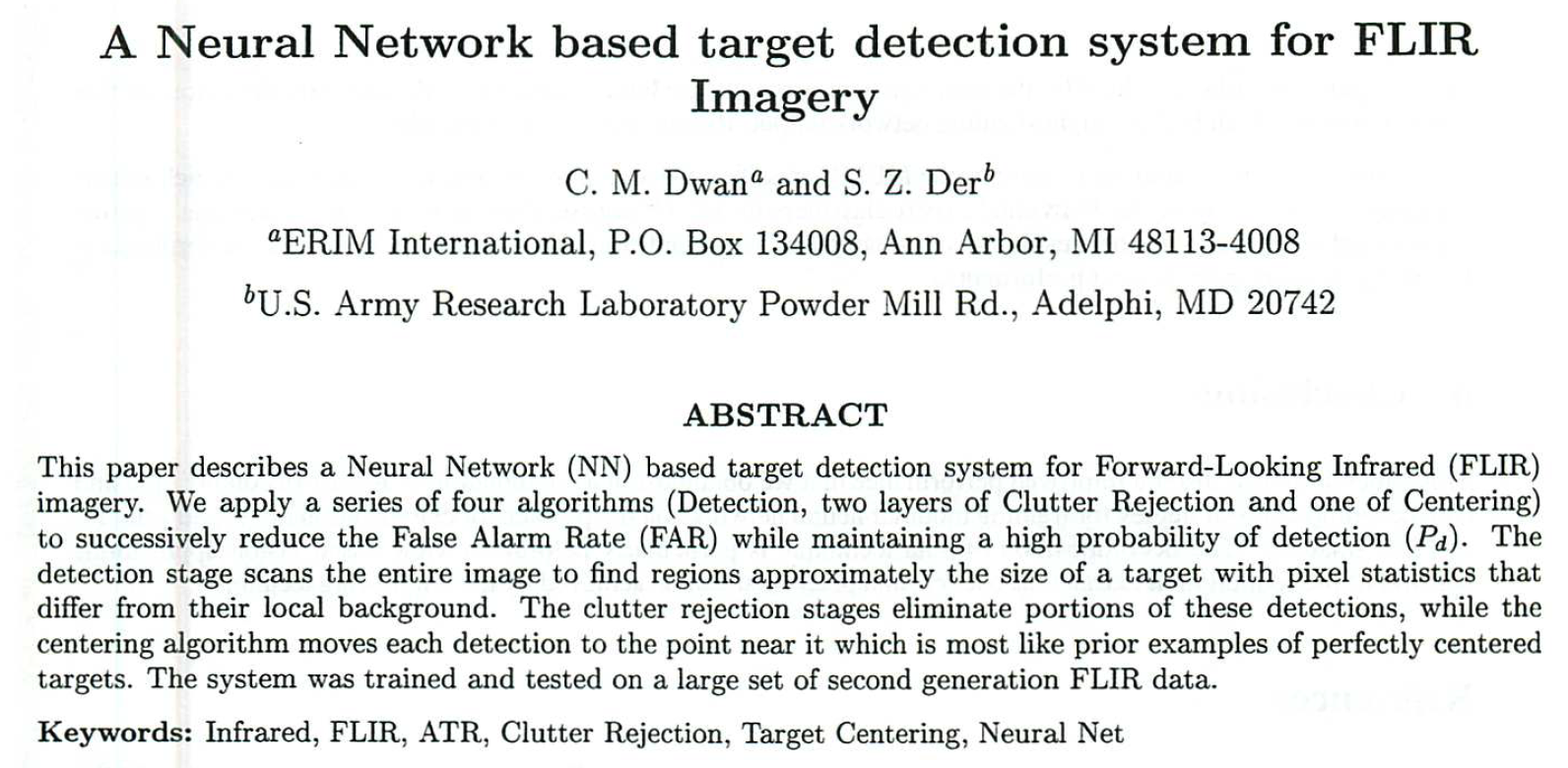
I’m thrilled that we finally have the data, the algorithms, and most of all the focus and discipline to bring AI to bear on human health. Practically speaking, I’m pivoting my professional practice to preferentially engage with the projects and organizations centering AI in the context of large, well curated, cross-domain datasets. That last bit – about the data being well curated and cross-domain is important because AI is fundamentally a data play. The emerging success of AI in our field is made possible by decades of work creating data platforms (warehouses, lakes, grids, commons, and all the rest).
Domain Expertise Matters
In the early 2000s, I was one of those young technology hotshots who thought that the key to biology was to be found in some clever algorithm. It didn’t take very many years supporting real biology before I abandoned that idea entirely and focused on learning enough science to not embarrass myself in lab meetings any more.
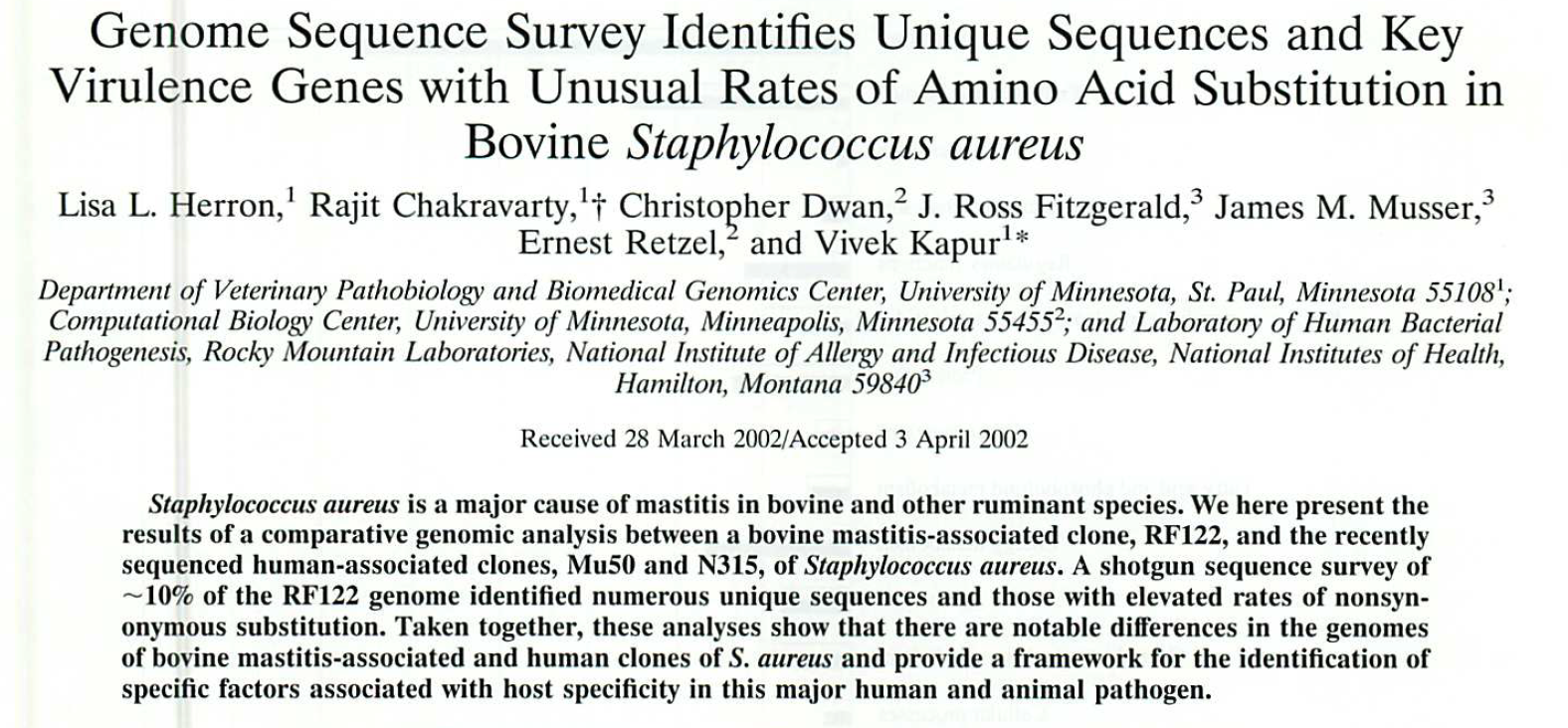
It’s hard to overstate the importance of domain expertise and data hygiene as we turn powerful algorithms and tools loose on large amounts of data. Recent history is littered with humiliating racist gaffes by companies who should have known better. Indeed, genomics is only slowly coming to grips with the fact that our datasets and early discoveries were built on a foundation of bias.
All of that is to say, “Garbage In / Garbage Out” still holds true even in the era of AI – as does “buyer beware.” Folks who carelessly fling petabyte scaled S3 buckets through a meat grinder / laundry list of algorithms … well … they will get what you might expect. It’s the same mistake I made early on, failure to respect the level of knowledge, context, and expertise encoded in seemingly simple concepts like a “gene” or a “diagnosis.”
If you’re ready to leverage those silos of data to power breakthroughs – whether it’s for early stage compound development, incorporating EMR and RWE for clinical decision support, or for getting over that last-mile into standard of care clinical use use: Give me a call, I would love to work with you, and I will prioritize engagements that support this timely and important mission.